Here is an extract from my recent eBook, Thirteen Short Chapters on Remote Sensing. It is the first part of “Chapter 4: Two things that can give remote sensing a bad name”. I thought it contentious enough to post as a blog entry to generate a bit of discussion. It also links back to previous discussions, such as this post on radar backscatter-biomass studies, or this one on understanding the backscatter-biomass relationship.
The book is available in Kindle format from Amazon or as an iBook from Apple. (It is best that you search for this title rather than giving a link, as it is region-specific, but this is the UK link for Amazon and this is the UK link for an iBook ; and I think this is the US link for the Kindle and US link for iBook. You don’t need a Kindle to read it; you can download various free Kindle readers from Amazon so you can read it on your iOS, Android or PC/Mac desktops.
I have noticed two trends over the years that I believe are misrepresentations of remote sensing: (1) not going further than using correlation statistics as the basis of understanding a measurement, and, (2) defaulting to discrete image classification as an “off-the-shelf” approach to understanding image data. Both are commonly portrayed as central tenets of remote sensing in books and webpages on the subject, and certainly a visit to many remote sensing conferences would make you think that these activities are remote sensing.
The use of correlation statistics is an interesting case. The approach seems to go like this: measure some biophysical property on the ground or in the atmosphere; then measure some data from an aircraft or satellite instrument; finally, draw a graph of one against the other and work out the correlation statistics. Apply correlation function widely and claim “retrieval” of physical properties of the Earth’s surface.
From some conference presentations, and indeed some peer-reviewed articles, you might think that this was the limit of remote sensing. I would argue that correlation statistics is just the stating point of your inquiry – they merely tell you that there is an association between the two parameters. It can be used to help formulate a theory about what is going on, which can then be tested, or it can be used to help test an existing theory or model. In themselves, the statistics are limited. Sure, you can use them in whatever operational tool you care to imagine, for the statistical correlations can always be checked and verified to your requirements using more statistics. But in themselves, they don’t tell you what is going on. You should use this observed association to develop a model of what you think is happening. This Forward Model (see Chapter 5) encapsulates all your knowledge about the subject, even if very limited. This will usually be a physical model. It seems to be fairly common for people to refer to “modelling” an observation, when all they are doing is representing descriptive statistics in a regression equation. You should at least qualify it with “empirical” before the word “model”, if you use this approach.
A neat example of why statistics alone can be problematic is given by Anscombe’s Quartet. This is a set of four sets of data points that when plotted look like this:
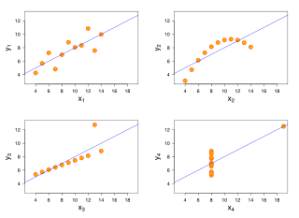
The importance of these graphs is that they demonstrate how recourse to simple statistics alone without a visual assessment (or more elaborate statistical analysis) may lead to erroneous conclusions: all four graphs have the same mean (9 for x, 7.5 for y), R2-value (0.67) and least-squared best-fit linear equation. Always remember these graphs when you are looking at your own data, or evaluating data from others. Certainly always look at the data and do not default to the statistical parameters alone.
Anscombe’s Quartet certainly illustrates nicely why statistics is not a substitute for understanding. This is the reason why I would argue that a physical model is important – with a model you can start to actually understand what is going on, or at the very least highlight the fact that you don’t understand it (which is also important to know). With a Forward Model you can change the observation parameters and determine if you can improve the measurement. You can predict what will happen with different instruments under different conditions. And as a consequence, you can test those predictions and modify your model accordingly. Sometimes it is certainly true that the physics of the observation or the complexity of the target itself defies a rigorous physical model, but the notion that this should be your ultimate aim should always be factored into remote sensing research. To stop at the correlation statistics is at best limiting, and at worst, foolhardy.
My second bugbear is classification. Let me first quote from Charles Fort, humorist, observer of strange phenomenon and creator of the word teleportation:
“In days of yore, when I was an especially bad young one, punishment was having to go to the store, Saturdays, and work. I had to scrape off labels of other dealers’ canned goods, and paste on my parents’ label. […]
“One time I had pyramids of canned goods, containing a variety of fruits and vegetables. But I had used all except peach labels. I pasted the peach label on peach cans, and then came to apricots. Well, aren’t apricots peaches? And there are plums that are virtually apricots. I went on, either mischievously, or scientifically, pasting the peach labels on cans of plums, cherries, string beans, succotash. I can’t quite define my motive, because to this day it has not been decided whether I am a humorist or a scientist. I think that it was mischief, but, as we go along, there will come a more respectful recognition that also it was a scientific procedure.”
From “Wild Talents”, by Charles Fort, p. 8, Redwood Books, 1998.
The obsession that remote sensing scientists have with classifying imagery of the Earth’s surface is much like Charles Fort and his can labels – the labels become the task, not the tool. Personally I blame the Landsat programme. The very first land applications of spaceborne satellites were based on …
[END OF EXTRACT]
You can buy the complete book from Amazon. (It is best that you search for this title rather than giving a link, as it is region-specific, but this is the UK link and I think this is the US link).
Yes, correlation is a problem! See for example the furore regarding NIR albedo and Nitrogen (there’s a nice write-up on the main paper on doi: 10.1073/pnas.1219393110, but also see the correspondence).
Ultimately, having a phyisical model (or understanding) allows one to learn from other experiments: the application of Bayes’ Rule is then straightforward and useful. Correlation studies tend not to be amenable to this “standing of the shoulders of giants” improvements.
Having said that, sometimes correlations point to areas where we lack the understanding to explain them out. You hope that a sensible model would be able to explain the correlations you observe. If it doesn’t, does that mean that your model needs to be modified?
The problem is correlation is a parametric statistic that assumes the underlying distributions are normal. One should make sure that the parametric assumptions are met before calculating a correlation. There are non-parametric measures of association that can be used when the parametric assumptions are not met.
One of the problems with the way classification is used in remote sensing is that there is a spatial autocorrelation between adjacent pixels. Parametric classification routines assume that the training measures are independent samples. This is not the case when adjacent pixels are used to characterize a land cover type for example. The problem is modeling this autocorrelation can also remove the information needed to characterize a land cover type or some other set of classes one is trying to partition an image into.
Reblogged this on Carbomap news and commented:
Extract from new book by Carbomap CEO Iain Woodhouse: